Transcript below
View Video
Listen to Podcast
Like podcasts? Find our full archive here or anywhere you listen to podcasts: search Community IT Innovators Nonprofit Technology Topics on Apple, Spotify, Google, Stitcher, Pandora, and more. Or ask your smart speaker.
Presented in partnership with Build Consulting.
Many nonprofit staff members feel frustrated with data quality problems within their organizations. Are you challenged with data accuracy, incomplete information, lack of integration between systems? Data quality defines your organization and can make or break your fundraising.
We often feel powerless to address these data quality challenges, in part because simply having the conversation is difficult.
- What is “data quality?”
- What can we do about it? Where do we start on such a big project that touches so many staff?
In this webinar, Build Consulting expert Peter Mirus explores the crucial aspects of data quality at your nonprofit: completeness, validity and accuracy, consistency, timeliness, and integrity.
Peter uses real-life examples from the nonprofit and public sectors to show how good data quality can have big fundraising and program impacts. In the second half of his presentation he follows up on these examples and explores the role of good data at your organization, provides tips on how to get everyone at your organization on the same page, and gives ideas on how to prioritize data for quality improvement.
Don’t miss this chance to learn more on how your organization can create and leverage better data to improve your fundraising and program results!
There was a 15 minute Q&A following the webinar, and as with all our webinars, this presentation is appropriate for an audience of varied IT experience.
Presenter:
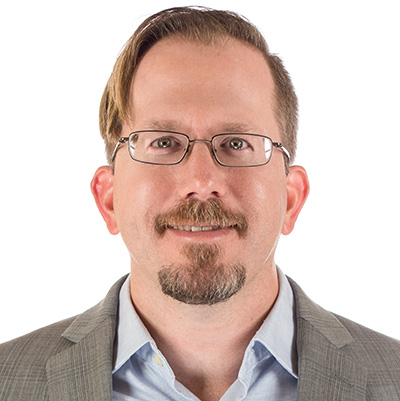
Peter Mirus co-founded Build Consulting in 2015, following over 15 years of nonprofit consulting experience in the areas of technology, branding, marketing, and development. Peter’s work for Build clients has a broad focus spanning many operational areas, including fundraising, program monitoring and evaluation, accounting, and impact reporting/analysis.
Transcription
Peter Mirus: Hello everyone and welcome to the Community IT and Build Consulting Webinar for November 2019, titled How Data Quality Defines your Organization.
Today I’ll be introducing a simple framework for understanding data quality that you can use to help drive data quality conversations at your organizations leading to improvements in the quality of your data as well as fundraising outcomes and mission impact.
Before we get started on the webinar, here are a few quick housekeeping notes: Don’t forget you can ask questions using the available questions feature and go to webinar. There will be some time reserved for Q&A at the end of the presentation. Avoid multitasking – you may just miss the best part of the show and as always, links to the recordings now available in both video and mp3 format on the Build Consulting website will be shared via email with all registrants after the webinar.
And just a quick note, we have another upcoming webinar from Community IT, it’s on Wednesday next week, titled Five Security Tips to Protect your Login Credentials and More and I’m told there will be a special offer for attendees during the webinar, so you don’t want to miss that.
A little bit of information quickly about Community IT and Build Consulting. We are two organizations that work exclusively with nonprofit organizations around their technology needs, serving over more than 1000 nonprofits cumulatively. We help our clients make strategic IT and Information Systems decisions that support the mission.
Whereas Community IT is more focused on Managed IT, Build Consulting is more focused on data management and information systems. And we have a collaborative approach that empowers our clients to make informed decisions for their organizations.
As I said, Build Consulting is more focused on data management and information systems and these are the services that we offer:
- Assessments and roadmaps,
- software selections,
- support for implementations,
- serving clients in terms of helping them to engage with vendors and manage projects and perform change management effectively.
- We serve as interim or part time CIOs for nonprofit organizations and
- we also provide outsourced CRM, data management.
All of our services are designed to help clients transform themselves to better serve constituents of all types, including funders, donors, program beneficiaries, even staff, volunteers, board and committee members, and of course, the general public.
My name is Peter Mirus; I’m a partner of Build Consulting. I have 20 years serving all manner of nonprofit organizations ranging in size from small to local–small and local to enterprise global-sized organization across a wide variety of industry categories and mission orientations, and over the past eight years I’ve worked exclusively with nonprofit organizations. I’ve worked with over 100 clients in the nonprofit, government and for profit spaces and I have three primary areas of expertise, information strategy in general, constituent relationship management and communications, and I’m Build’s resident expert on data quality.
Build Consulting was founded with the belief that tomorrow’s best nonprofits will use technology to transform themselves and the world. However, we were also looking at the industry statistics when we started back in 2015, that more than 50% of nonprofit technology projects fail, and on analysis, the reason that the technology moves–the reason for this failure rate is that the technology moves forward, but the organization does not. In today’s day and age, there’s most often a good technology or at least the sufficient technology solution for most nonprofit needs and there’s something else, not the system, particularly, but other challenges that are leading to lack of having maximum effectiveness with chosen technology solutions. We often present this formula to our clients, it stands for old organization plus new technology equals expensive old organization and this is just to underscore that transformation in technology projects is critical to your success. There’s no such thing as a technology change project that isn’t also to some degree an organizational change effort. To acknowledge that we built something called the Information Strategy Framework, which evaluates nonprofit organizations as pertaining to their data and technology needs through the lens of leadership and governance, operational capacity focusing a good deal on project or program management and change management, process and requirements, documentation, data modeling, and then finally, technology.
And technology is intentionally placed last in this framework because it really underscores that unless you get everything upstream right of selecting and implementing the technology, you have a much greater chance of–or risk of failure without technology implementation. And today, we’re putting data squarely in the crosshairs of the conversation, as we talked about data quality and that leads us into our agenda for today. (5:24)
I’m going to quickly talk about some of Build Consulting’s observations regarding data quality in the nonprofit space and briefly talk about making the case for data quality so you can help introduce the conversation of “Why data quality?” at your organization.
We’re going to talk in a little bit more technical terms about the five dimensions of data quality with some company and case studies.
And then I’ll provide some practical recommendations that you can use to help your organizations think about data quality and prioritizing what data to collect and maintain. And finally, hopefully we’ll have some time left for Q&A at the end.
We had over 90 registrants for this webinar from a wide variety of nonprofit organizations throughout the United States and Canada and I see that currently 22 of those registrants are currently attending. Thank you so much for being with us today to talk about this topic. As I said, I hope we’ll have some time remaining at the end of the presentation for questions and answers, but if you asked a question during the registration process or during today’s presentation that remains unaddressed by the end of today’s session, my personal contact information will be provided immediately prior to the Q&A portion. So please feel free to reach out to me directly with a follow up if need be, I’m happy to interact with you.
So now we’re going to get started into the meat of the topic. (6:50) Build Consulting’s been in a lot of conference rooms. We’ve been through multiple information system design, implementation, integration, and migration projects, and we’ve seen social good organizations encounter and then deal with or fail to deal with data quality issues, and the kinds of decisions they make regarding data quality are interesting to us in three different ways.
First, data quality decisions have the ability to determine how successful an organization will be, how it sets itself up for success, mediocrity or failure based on its ability to manage and leverage its data to make informed business decisions and also drive impact.
Second, data quality decisions are profoundly revealing of the strengths and limitations of an organization. Does the organization have the strategic vision to make appropriate investments in data quality? Does it know what relationships the organization has and what data is profoundly important to nurturing and growing those relationships? And does it have the leadership and discipline to require staff to adhere to corporate policies, standards, and business processes those results in good data quality?
And third, many organizations don’t fully comprehend the picture of what data quality is or what data is.
Data is all the information that your organization collects. It’s both qualitative and quantitative, both structured and unstructured or in another way of putting it both the narrative and the numbers.
So how do we make the case for data quality?
I’m going to give you one example of how to do that within your organization. This is an aerial photo of the Washington Navy Yard, which may be familiar to some in the Washington DC area; it’s near where the Washington Nationals stadium is. It’s the oldest shore establishment of the US Navy and the photo you see here was taken in 1985 and superficially, it appears to be somewhat like, a prosaic urban landscape as these things go.
In 1980, Congress created the Super Fund to pay for the cleanup of the country’s most hazardous waste sites and what a lot of people don’t know is in 1998, the EPA put the Washington Navy Yard on the national priorities list, one of the 1700 prioritized cleanup projects of the 47,000 waste sites in the nation. This site today has a status of “active,” meaning that cleanup facilities have not yet been completed. The Anacostia River on which the Navy Yard is situated is part of a complex ecosystem, about which much data has been collected, but about which there is still much to be learned.
In data quality, we talk a lot about the real world object. Data exists to describe a real world object, the better the quality of your data, the better you are able to understand the real world object, and hence, your relationship to it, how your actions impact it. Just as the river flows continuously, sometimes the real world object is in a constant state of flux, and then it becomes more challenging to maintain accurate data.
Good data quality has been and continues to be part of the Anacostia watershed cleanup process, which has seen participation from numerous organizations. Gathering and integrating high quality data from various sources is critical to the work of those organizations, sent to other organizations, both large and small, participating in conservation efforts worldwide, such as those impacting the Washington Navy Yard and the Anacostia watershed adjacent.
Whether removing contaminants from the Washington Navy Yard in the Anacostia watershed, running medical field trials in Africa, training small businesses in Afghanistan, empowering women and girls in Eastern Europe or combating domestic violence in the United States, the information you gather about the people you serve and their local environment has a good deal to do with the impact of your efforts. With so much data lacking that we wish we could obtain, the quality of the data that we do have to describe those real world objects becomes of paramount importance.
Data Quality directly helps to create life quality. (11:07) Data Quality is not just about accuracy, as we will see in the upcoming parts of the presentation, It’s multi-dimensional. We need to place the right information in the hands of people at all levels of our organizations, from the C suite to the frontline staff to the constituents. This will help to create greater efficiency and impact. By doing this, how can we help people do new and amazing things? Those are the questions that we should be asking ourselves.
And finally, the data that you have enables you to have a voice to be heard. You want to get through to people. The stories that you tell with the data that you have creates intellectual and emotional resonance with your key audiences. It communicates the value of your work and helps to secure the funds and influence necessary to do the work well. So having good data and easy access to the data, and translating the data is very important to telling a compelling story about your organization and the needs of its constituents and beneficiaries.
Today for the sake of simplicity, we’re going to talk about five dimensions of data quality. Those who are familiar with data quality terminology as used by such major funders as USAID, will recognize some points of familiarity and some differences.
(12:24) The dimensions that we’re going to cover today are
- completeness,
- validity and accuracy which are distinct concepts, but well paired together,
- consistency,
- timeliness, and
- integrity
And we’re going to take each one of these in turn, talk a little bit about what it is, and then provide a case story example of how it’s important.
When data is complete, it has all of the necessary appropriate parts.
We use the terms breadth and depth to describe these qualities of completeness. When we say breadth, we are asking does the data have all of the expected attributes? Think of these attributes as columns in a spreadsheet and when we say depth we’re asking, is the data set is fully populated as expected? Think about the rows in the spreadsheet. To provide a very simple illustration, when gathering constituent information you do not have complete addresses if you’re not capturing the state in which each constituent lives. The fact that you have a field or column of data to capture the state speaks to the completeness of breadth within the data set. The fact that you have a value in that column for each of the constituents is indicative of the depth of the data.
Let’s say that your organization is running an economic development program in Afghanistan, focused on training small business owners and entrepreneurs to engage in the global economy. As an aspect of data collection, the program managers and chiefs of party are tasked with collecting information on women serve through these programs, ann imperative coming from both the funder and your own organization. The program is executed through multiple technical assistance projects out of various locations within Afghanistan, and the data collection is being done on paper and then entered into spreadsheets. However, the data collection method was not standardized and some projects had a field to capture beneficiary gender and some did not. Some of the projects had agenda field, but did not consistently manage the paper documents to capture that information resulting in loss of backup information and contributing to data gaps. The problems within this scenario impact both the breadth and depth of the information. The data was not complete and in order to successfully provide reports based on the data, the program managers had to make best guesses to fill in the information. This impacted the data quality and the trustworthiness of that data.
This is the kind of completeness problem that you want to avoid. You want to make sure the gender field is available as an attribute of the object you want to describe and you want to make sure that the field has information in it. This is a simple example of the problems that can be created by incomplete data. When a major funder like USAID loses confidence in the data quality and trustworthiness of the data because they see problems, they lose confidence in your ability to deliver on the program and the flip side having good data quality and backup for that data causes the funder to gain confidence in the program and increases the chances the program will be expanded or renewed. Completing this is one of the easiest concepts to grasp and this next one is fairly easy too, although requires a little bit more explanation.
So let’s talk a little bit about data quality: validity and accuracy.
In the world of data management, including performance data management, validity is sometimes called the conformity to a domain of values. That domain of values might be an actual set of values such as a list of states, a range of values, such as a number between 1 and 100 or a rule that would generate a value such as GPS coordinate fields being automatically populated from an address. In other words, validity is judged by comparing data to criterion or constraints. So in order to test and measure validity, you need to know the values or rules to which the data should be compared. These are the rules that prevent invalid data from being entered, which goes a long way to help avoid inaccuracy. You can extend the definition of validity to create rules for preventing or assessing duplicate data, which some of our registrants asked about, which in data sets over a very small size is difficult to assess visually. For example, you can create a rule for a beneficiary database, indicating that it should be impossible to have two records with the same last name and street address, you can be using a piece of valid data that is nonetheless inaccurate. In order to examine accuracy, you must examine the real world objects that I mentioned before and compare it to the data. So for example, if I was filling in a state field and entering MD from Maryland, which is a valid response, but I lived in Virginia, the data would be valid but not accurate.
Here’s another real world example from my past client experience about how data validity and accuracy is important. Last week this man gave was a 10,000 dollar per year giver to your organization and this week, he’s no longer your donor. Why? This man’s wife was on your mailing list. Some donations were made in her name and some in his, then his wife died. The man let you know that his wife was deceased and a development person at your organization assessed the woman’s record and selected the wife’s status as inactive deceased from a drop down list. The drop down list ensured that the person could only select from a range of values. Therefore it was impossible for the entry to be invalid and in the donor database; the information also accurately reflected the real world situation. So to start out, we have both validity and accuracy in the record. However, because of poor data integration between the donor database and the mailing list, the wife was never taken off the organization’s mailing list. In that separate data set she continued to have a status of active. Therefore, even though the husband notified the organization several times that his wife was deceased, the organization continued to send mailings to her. The person doing that data entry into the donor database reported the problem to a manager, but it was not red flagged as a potential persistent data quality issue. As it turned out, the problem took only 1,000 dollars to resolve in the integration by tweaking it. But by the time the organization recognized and reacted to the data quality problem, resulting in inaccuracy in one part of the data set, the man was emotionally upset and had lost confidence in the competency of the organization. He therefore cancelled his pledge and began giving the same amount to another organization. And so how does this translate financially? For lack of a 1,000 dollar data quality decision being made proactively, the organization lost 200,000 dollars in potential revenue or what it expected to get from this donor during his lifetime.
So that covers validity and accuracy.
Now we’re going to talk a little bit about data quality: consistency (19:12).
This is a little bit harder for organizations to think about and it requires a lot of discipline to do well. Data consistency is insured by the methodology applied for data collection remaining consistent over time, and it is measured by the degree of similarity or the absence of variety within the data.
We can measure consistency of similar data over time by looking for trends and differences from the trend. Consistency is what allows for the authentic analysis or interpretation of data over time and across space. In order to ensure this as possible, the data collection process must be well documented, (which a lot of organizations don’t do, unfortunately) and standardized (also, something a lot of orgs don’t do) to the fullest extent possible.
So for example, considering every program within a vacuum can be deadly from the standpoint of organizational indicators, the aggregated will provide performance data to both the organization and its key funders, as well as to industry analysts and influencers. If the consistent collection rules for organizational indicators are not applied across all programs, then the organization’s performance may be off in ways that are very difficult to spot just by looking at the data absence the ability to evaluate the record of different data collection methodology, so make appropriate corrections – and that’s a lot of quick technical speak, which hopefully the case study will be successful in resolving if you are a little bit confused. I confused myself just by narrating that.
So in the case of this story, we are going to talk about consistency and Youth Services (20:53). Consistency will frequently be a problem when critical business definitions change over time, but the fact of that change is not recorded as a separate data point that can be applied to help interpret data trends. So for example, an organization’s definition of “at risk youth” might change over the course of a prolonged period of time, and data might be collected relative to that definition. This means that there was a lack of consistency within the data collection and hence, within the data itself. The fact of the definition changing is a critical piece of data necessary to interpret the lack of consistency within the data sets and make adjustments accordingly when doing the analysis. Otherwise, it might seem as if the real world objects, in this case the number of at risk youth, did change, but in actual fact, what changed was how the organization defines, “at risk.” And now imagine if the organization had changed the definition of “at risk” and also the definition of “youth” in terms of an age number or age range, and had not recorded that fact, say they had done each of these three and five years ago respectively, and they’re looking at their service metrics over a period of time and trying to figure out exactly what happened in the data to indicate the trend of differences when in reality, they weren’t able because of lack of historic record to determine when the collection methodology had changed or the definitions had changed.
Now we’re going to talk a little bit about data quality: timeliness (22:29).
Timeliness refers to the regularity of the data collection and the availability of that data to decision makers which are two distinct things. But the overall goal is to decrease the time between when the real world object changes and when the change is known by all relevant parties, which could include program staff, organizational strategists, funders, and other information consumers.
Important points to consider regarding timeliness are as follows:
- If the data has a high degree of volatility, there is an increased risk that the data will not meet standards for timeliness. Volatility is the degree to which data is likely to change over time. An example of low volatility data is gender. An example of high volatility data is salary. Data that has high volatility will need to be checked more frequently to ensure that it remains timely.
- Data fails to be timely if there is a lag between when a factor becomes known and when it becomes available for use. An example of that is if a program staff or in the field becomes aware of a change to a real world object, that is important to program outcomes. But if that person then fails to communicate it to program decision makers in a timely manner, perhaps because the change occurred between regular reporting intervals like quarterly, it could be so that the information doesn’t arrive in a timely manner to make a decision and this highlights the need for all staff to follow up both the letter and the spirit of the law when collecting and transmitting data, because if you’re two and a half months out from a quarterly report, and you don’t take advantage of an ad hoc opportunity to kick data upstream or downstream as the case may be, then it might not be available in a timely manner to support a decision.
- And finally, data fails to be timely when there was a lag between when the data is updated at the source and when it becomes available to decision makers at the other end of the information chain. So for example, if data is contained in the spreadsheet at the program office, but is not yet transmitted HQ, then you have problems with timeliness and there are some subtle distinctions there that might be only apparent if you give this a second listen or if you have follow up questions, let me know.
So this is a picture of the devastation that occurred on the New Jersey coastline during Hurricane Sandy and many of you who are watching the news around that time will be familiar with images such as this particularly those of you that are local to that area. Timeliness is of critical importance particularly when it comes to disaster response. The timeliness of accurate data such as weather forecasting and resource availability and positioning helps governments and relief organizations to successfully deliver services to assist those in urgent need because of a natural disaster or in another example, some sort of mass human rights violation or civil strife. In the events leading up to, during and following a large scale event like Hurricane Sandy, the timeliness of data can make a huge difference to providing timely relief. You will find in these particular kinds of situations that the efficiency with which the organizations involved exchange information and the timeliness of that data being able to be combined and interpreted for various actions has a lot to do with how organizations and agencies fare both in the effectiveness of their activities and then also in the eyes of public perception and the assessment that takes place following the aftermath of an incident such as this.
Now we’re going to talk a little bit about data quality: integrity (26:05).
Data integrity is not so much the absence of corruption in the data, although when people think of data integrity, sometimes they use that, in that sense, or they use the word integrity in the sense of as synonymous with data quality. Data integrity and quality are sometimes talk about synonymously. But in this case, we’re going to define data integrity as how all of the parts fit together, how the pieces make one whole thing.
Some of the registrants for this webinar asked questions about having data in silos or how to integrate different data sources or how to make the case for consolidating all of their data into one location and there is a lot of cost value opportunities to be considered in making those kinds of decisions and gaining organizational momentum for them. But to get back to the idea of integrity as being all the parts fitting together, if you have a large database or data warehouse or even a file share with multiple Excel files containing records from a range of systems, people who are using that data have an expectation that they will be able to connect it. They will expect the data to be integrated, right? The integrity of the data, in this sense, is one of the first things people will question when they cannot use the data in ways that they expect, so it is important to have a plan for how you’re joining data from different data sets or tables, and how well the joint data fits together. When you have multiple information systems and the data within them relates to each other, you need to create a way to make sure the integrity of that data is present when it is related for the purposes of gaining insight and making decisions. And it was interesting, I was reading a report the other day that said that the number one challenge for business users of CRM platforms, or CRMs is integration issues. And this will be no surprise to the majority of you attending this webinar. Also it said that a majority of users would be willing to exchange some features for better ease of use with better integration being an ease of use factor, so I thought that was interesting.
At a very high level, measures of integrity are measures between different tables in the database, how you join data from different data sets or tables, and how well it fits together. It’s basically your ability to relate the data cohesively.
(28:34) This is a brief story about integrity and health care. Integrity matters in the healthcare industry when you need to be able to bring together data related to diseases from a wide variety of sources. Viewing all of the medical records from a particular hospital is relatively easy by comparison to collecting and analyzing disease related data from a variety of different hospital systems and other similar institutions. Without being able to easily integrate the various data sets, it is very time consuming to be able to analyze the data perhaps even to perform predictive analysis that will help to control the spread of a particular disease. So in this case, as in many cases, and including the last one around disaster relief, where there was a high degree of criticality associated with making a quick response, integrity is extremely important. The ease with which the different parts of the data fit together. And you’ll notice that there has been a lot of work done to standardize the collection of healthcare data within the United States and globally, particularly over the past decade. It is a continuing challenge and a continuing battle. One of the reasons why that work is being done is to ensure there is integrity across multiple different systems – across multiple different means of collecting data – and that eases the ways in which people can use that data to increase public health and mitigate risk and also just to treat individual patients within your community.
So to do a recap—a definitional recap—here are the aspects of data quality that we covered today (30:01):
- Completeness, which is the breadth and depth of the data as considered through the lens of gender equality was the case story that we used.
- Validity and accuracy, which we consider through the lens of donor retention.
- Consistency as considered through the lens of youth services.
- Timeliness as considered through the lens of disaster response and
- integrity as considered through the lens of healthcare.
So, again, this is a lot of information that I’m pushing out to you really fast and I just want to remind you that the information from this webinar will be available in several different formats after. By next week, it’ll be available in video and mp3 format, so you can watch it or listen to it on your commute and take notes and it also will be available sometime after that in transcript format, which we will also make available for you.
For each of the data dimensions covered in today’s presentation, we cited a real life example that included some sort of problem or challenge. One might reasonably ask how these challenges can be addressed? And this is the part where I get into some recommendations about how you can start advancing conversations about data quality within your organization because it’s sort of hard to get out there and say, “Hey, everybody, let’s talk about completeness, accuracy, and validity, you know, consistency.” all of the different, more technical definitions and the technical aspects of data quality.
So, the approach that I prefer is rooted in two principles:
One, there is no such thing as perfect data quality and I find that telling people this, it helps take the pressure off the conversation, because it grounds the following conversation in a realistic perspective. For data to be perfect, it would have to always be precisely representative of the real world object. And we know that that’s extremely difficult, if not impossible, for the objects we describe with data in the course of performing our work.
And the second perspective is that data is meaningless, absent the context of relationships. Your relationship to a real world object and the relative importance of that relationship compared to other relationships dictates your data quality priorities. This is what drives you to ask the critical questions; “How complete does my data need to be? How accurate does it need to be?” etc.
I often think about these things in terms of prioritizing the relationships that the organization has, and then what data you need to maintain your responsibilities inside of that relationship. So the highest priority is the data that you really need to maintain your responsibilities in those relationships. In contractual relationships that’s very easy to identify. But in other kinds of relationships, especially in a relationship between say, you and an aspect of the environment, it can be a little bit more difficult to determine, sort of “What is my obligation within this relationship and what data does that obligation necessitate that I collect and maintain as a matter of high priority?”
And then the next level of data that you should prioritize data quality for in all of its aspects, is that information that’s not necessary, but is there to allow you to operate further and analyze the situation, a set of conditions, and to see what opportunities are available based on that additional data.
So I would require that to be defined something more as opportunity data or speculative data.
And these are the kinds of conversations that you need to have and I find that people are much more open to talking about relationships and mission than they are about talking about data quality, per se.
So, the way to get into conversations about data quality is to say, “Hey, we’re not shooting for perfection here, but we’re shooting to get better, so that we can have better fundraising outcomes,” for example, or better mission impact for this program or that program. “What can we do about that? Well, let’s start with our relationships. Let’s start with mapping our constituent engagement life cycles. Let’s start talking about what our obligations are within these relationships, like how does our organization position or define those things?” And then use that to transition into a question about priority or key processes that take place within that constituent lifecycle or the life cycle of managing the data about that thing or executing processes? And then say, “What data do we need to collect as part of that process? And what sort of data quality rules do we need to build into it?” whether that’s from while the data is being collected or after the data is being collected and some sort of review process or how that data is maintained over a period of time.
So that’s the thing that I really want people to take away is that (35:00) data is meaningless, absolutely meaningless, absent the context of relationship. So, that’s the lens that is most successful in having these kinds of discussions.
This specific approach to data quality (35:17) for each organization therefore relies on clarity and strength in the organizational strategy both at a high level and as extended through programs and projects. The strategy should tell you the relationships most critical to success and the value placed on each relationship, then you can prioritize your resources accordingly to that value. This often requires some degree of transformation at an organizational level before improvements to technology may be effectively leveraged and that brings us back to this slide that you saw earlier, which is the same. When you’re talking about data quality and improving data quality, either inside or outside the context of technology, usually inside the context of technology, transformation is critical to your success and transformation effectively starts with leadership.
Pretty much every question that I get about data quality eventually traces its way back to leadership, to organizational strategic definition to really clarifying and/or understanding what relationships are high priority to the organization. There are ways that you can go about doing that or getting help doing that if that’s something that the organization requires additional perspective to do and Build Consulting helps organizations with their constituent or beneficiary relationship lifecycle definition is a key aspect of our work.
I think that it’s important to celebrate the successes (36:45) Organizations of any size and complexity that make data quality a cultural endeavor, typically have good data quality relative to their need for such while organizations that do not, will generally have poor data quality.
So do it well; have some enthusiasm for it. Try to inject enthusiasm about relationships into the discussion of data quality at your organizations and then make sure to celebrate your successes even if they’re incremental, even if they don’t get you all the way to where you want to be. For all the talk about data quality in the social sector, having truly high quality data is relatively rare and the ability to use that data then for a meaningful action is even rarer.
So if you are able to have successes in your organizations make sure that you celebrate them yourselves. Take a breath, pop the champagne, and try to get others excited about it, too. Because, in the absence of that exuberant spirit, focused on and contextualize within relationships has been the primary benefit. It’s hard to maintain data quality over a period of time. It really is a lot about turning data quality into a storytelling thing, into a relationship thing, into a celebrated thing inside of your organization.
(38:11) So again, I mentioned that this was a lot of information real fast.
I wouldn’t necessarily be able to answer all of the questions, although we do have some substantial period of time left for Q&A if there are any questions that people would like to discuss. But otherwise, you can connect to me via email, that’s my email address up on screen. Also, if you receive the newsletter for Build Consulting promoting this webinar, my email is the sender’s email for that, so you can respond directly to that email message if you still have it. You can reach out to me through my Linkedin profile at linkedin.com/in/petermirus or you can just visit our website at buildconsulting.com/contact.
The resources section of the site is where we post all of the content for these presentations afterwards. There’s a lot of good information there about information strategy, about Data Quality. Also in our blog, there’s a lot of information about leadership engagement and technology and data initiatives. There’s an information strategy white paper for you, so there’s a lot of great resources there that you can take advantage of and we’re constantly producing new blog posts and resources and webinars all the time. So please make sure to check that out on a regular basis and if you haven’t yet, while you’re on the site, take an opportunity to sign up for the newsletter as well.
Q&A
And now there’s an opportunity for me to answer your questions, if you have any, you can use the chat feature or the questions feature in Go To Webinar to ask questions, so I’m just going to hang out here and if you have any questions, please send them through.
(39:51) So one of our attendees asked, I’m curious and others may be too about what technology tools do you use or recommend to manage all the data?
That’s a great question. It’s such a broad question. There are so many different data management systems out there for various needs. There are so many different integration tools. There’s so many different data analysis or reporting tools. It’s really a difficult question to answer.
I’ve been working a lot with organizations over the last several years on consolidating data from a diversity of different points onto a CRM platform that can also be used, sort of, in the manner of a data warehouse and usually that means Salesforce or some other broadly capable CRM platform, such as Microsoft Dynamics CRM is increasingly becoming an option in that area as well. You can look at our Build website to get more information about some of those products and their evolution. But one of the things that we’re constantly talking about with our clients is, you know, what’s the benefit of what we would call a platform play for their organization. Do they have the organizational maturity and the resources to be able to pull all of that data together on one platform? or do they need to figure out how to keep it an individual, what we would consider, best of breed purpose-design solutions for particular needs? whether that be human services case management, or program management systems or what have you, and then, I figure out how to integrate and then report on that data.
Another thing that I’ve been working on a lot lately is integrating either one way or two way data syncs between Salesforce, as an example of CRM system, and Intact or another ERP system, to really make it possible for program teams to be able to see data from different areas of their organization. So, program budgets and finances and also program reports and workflow and processes. And there’s a lot of different ways that you can do two way data syncs between the systems to say, “okay, well, the program teams need to have access to this data – some of its financial, some of its not – they’re only going to be accessing the data through Salesforce, but they’ll still be able to see it.” And then similar for the financial folks, they’ll be able to have access to some programmatic data regarding milestones and reporting, that’s non-financial in nature, but also be able to get the full financial details and they’re only going to be doing that through the ERP system, and then having some collaboration tools that consolidate across the two. So that’s a rambling answer, but it’s just talking about some things that I’ve been doing lately.
(42:40) Another user asked, How do I, as a Data Quality Manager, address the different volatility required by some data? Are there specific techniques as compared to standard data management? I’m intrigued by allowing for this elasticity of data.
I’m not sure that I understand the question entirely. I think the main thing that I’ve encountered just in general is for organizations to call out high volatility data and really classify data as to its degree of volatility, which might be done on a high, medium or low level of volatility, and then how often that real world object needs to be reconsidered, maybe through a survey, or some other sort of observational method to determine whether that data has changed. And really maintaining those schedules and milestones and giving yourself the tools in order to do the trend analysis. So, it really comes back to, for high volatility data, it comes down to the frequency with which you revisit the real world object to make sure that the data remains accurate, and having a system that allows you to maintain the record of history on that data point and also allows you access to that history to be able to do comparative reporting or trend reporting. So, that’s all I have for you right now in answer to that question, and I’m happy to follow up with you via email.
(44:15) Somebody asked regarding consistency, how to create and implement best practices, how to encourage adoption? That’s a great question.
I think it starts primarily with just awareness. A lot of organizations don’t maintain change logs in regards to policy and procedures and also, technology as it pertains to data management. Data gathering as being a part of data management and then data management over time, so it’s really just necessary to say, who owns this data? in this particular area of the organization and as a part of that ownership, what are they responsible for maintaining in terms of change logging? In absence of that it’s difficult to say, you know, in change logging should be done not just in regards to when policies change, when practices change, but also in regards to when major data set changes were made.
So as an example, if you did a data cleanup initiative in November 2019, and remove 20,000, duplicate records from a constituent database of 300,000 total records and you didn’t make a note of that fact, three years down the line, if you’re looking at it, you might say, “Well, we took a dip of 20,000 constituents in that year, so did we have a bad year or did something else happen where we made some sort of compression to our data?” and if you didn’t have a historical log that allowed you to interpret that data point, and mark it in some sort of visual or narrative fashion for people that were translating the data, you wouldn’t be able to make that determination. I would say best practices, just make sure that people slow down enough to develop the policies and procedures, and be aware of when they’re changing something that’s going to impact data and then put it in a well formatted, historic log. And this is a practice that I’ve instituted at several different nonprofit organizations and it really takes discipline and it really takes the organization understanding the time commitment that’s involved in that, especially if there are frequent changes, to make sure that it works properly for the entire organization.
(46:35) Another person asked, What about more affordable options for small nonprofits? That’s a great, great question. There are a lot of options out there for small nonprofits and I’d really need to know more specifics about what the needs were in order to answer that question. Nonprofits–smaller nonprofits often have challenges in that, if they go for an all in one system, it can be really expensive, or it can require a lot of in-house expertise to be able to manage it well and keep it configured and maintained, even if it’s a cloud based SAS system. But they sacrifice data and integrity if they go for a bunch of more loosely connected or integrated small systems that are more in those best of breed categories that have lower costs, so there are just some trade-offs there and it’s unique to every organization.
(47:36) Somebody asked, I’m trying to develop a data management plan to streamline data processes, improve data quality, integrate all internal data reports, as well as external databases… (That sounds like a big task!) … like community needs data, elevate data utility and save time and data analytics, know of any templates or tools to help simplify, unify, and enhance our data management. Wow, that’s a big question; it also says it includes program impact data fundraising and donor data, community data, administrative and financial data, etc.
Wow, I do have a template that’s available. It’s not right for every organization. It’s–we end up approaching this in different ways depending on the capacities and the sort of maturity of each organization as it comes to data or information management because, if you communicate something that you expect to be broadly absorbed and followed in highly technical ways, when there isn’t that same language compatibility, you’re just going to shoot yourself in the foot every time. But I do have a template that is for information management policies and practices, helps identify domains of data ownership, and helps manage everything from data quality to data security, and helps identify policies, procedures, accountability measures, all that kind of stuff. Again, it’s really particular and how its applied to each organization and it’s often most effectively done in one particular use case inside of an organization, make a success story out of it, and then move on to the next area. So if you’d like to follow up with me on that, I can make that available to you. I’m not sure how useful it will be as it currently stands, because it’s pretty generic, but it’s definitely even just a quick run through of the sections of that document will provide some meaningful perspective, so I’m happy to do that, only take a few minutes.
(49:43) Any resources to access about data volatility? that was another question. I don’t know what exactly is meant by that, if it’s pertinent to–in terms of templates to plan for measuring data volatility or to help create plans for monitoring highly volatile data, that’s one aspect of what that question could mean and another could be just are there any articles that have been written about data volatility?
The answer to those questions are yes, those things aren’t things that are directly available from Build right now, but they may be over time, but there are program impact evaluation type resources online that you can find that will help talk to some extent about data volatility and how to address that within your programs mostly from data collection methods standpoint and from a performance or impact monitoring and evaluation standpoint.
(50:50) Another person asks: Management does not seem to value the direct data management team efforts and successes it is all about the core business, legal services in our organizations case, what are your recommendations for catching their attention? That can be hard. I think the case for change is often predicated on doing two things. One: it’s quantifying, to the extent that you can, the business opportunity to be gained by having better data management, like what story can you tell about the opportunities and how can you make that as quantifiable as possible? Another one is to take, which is sort of less favorite, is to take the risk assessment approach, like what risk does it take, put the organization in terms of maintaining its fiduciary responsibilities or in terms of not being able to tell a story effectively or in terms of just pain and suffering or morale that were reduced in high turnover because of the data management challenges inside of the organization, those are all things that you can do for catching the attention of management or executive leadership.
Ultimately, another way to do it is if the organization has a broader need for just some CIO level perspective, you can introduce that idea; it might be of broader benefit to the organization across a wide range of technology and data projects, or even just from a business analysis capacity perspective.
So, you could make the case for either CIO mindset training for executives or management teams, or even individuals. That’s something that we can provide on an as-needed basis from Build Consulting. We have a little training program for that that we don’t publicize, but we do offer and use from time to time. Or we could talk about the different business benefits of introducing part time or interim CIO into the organization and include among those considerations, Data Quality and the benefits of better Data Quality to the organization in general and how to make that argument, so I hope that’s helpful.
I’m open to having a further dialogue about that as well. I’ve had trouble specifically with that in the legal services space as well, so it’s a challenge that’s familiar to me and I’m sure we could share war stories about it.
There aren’t any other new questions coming in, but I just would like to say, in closing for this Q&A section that there were a number of questions from registrants as I alluded to earlier, about specific systems, about integration potential between different specific systems.
This isn’t really the forum to address those questions and, in a lot of the cases, I would probably need to ask follow up questions to get the sense of what was specifically needed.
(54:00) But I will just chime in and say there was one specific question about the Raiser’s Edge and maintaining data quality in the Raiser’s Edge. There are tools that are available for that, that are both baked into the Raiser’s Edge and are available as third party standalone tools, particularly from Omatic. And in terms of standardizing, somebody asked a question about standardizing, centralizing all data, and the Raiser’s Edge that may or may not be a good solution. Raiser’s Edge really does what it does very well, but it’s ill fit for a number of other things, which is why organizations that have fundraising and service delivery aspects that work or fundraising from donors and membership might use two different CRMs, of which Raiser’s Edge might be one, and integrate the data as needed. Integrations can also be a challenge with Raiser’s Edge but they are possible. So that’s a broader conversation, so if you have any Raiser’s Edge specific question, we do have a lot of depth of experience in Raiser’s Edge due to its prevalence within the nonprofit space and are frequently running up against the client, so that is something we’re happy to get into with you on a more specific basis and I can either do that or refer the question to one of my colleagues.
And finally, somebody asked if I could put my contact information back up on screen. Here it is [email protected]. linkedin.com/in/petermirus or simply use the contact form on the website.
Again, thank you so much to everyone who participated today. If you want to revisit any parts of this conversation an email will go out to all registrants notifying them when the video on YouTube and the mp3 file will become available. And in addition to that, as I said, usually about a week behind that, the transcript is available. The transcript is available on the same page as the recordings are posted, so it’s the kind of thing where, if you email me and ask me to let you know when the transcript is made available, I’ll be happy to follow up with you on an individual basis or if you just check that page about a week following today’s date, it’ll actually be there.
So again, thanks to everyone. It was great spending this time with you. I hope it was helpful and have a great rest of your day, bye.