What does your nonprofit need to know about AI and ethics?
View Video
Listen to Podcast
Like podcasts? Find our full archive here or anywhere you listen to podcasts: search Community IT Innovators Nonprofit Technology Topics on Apple, Google, Stitcher, Pandora, and more. Or ask your smart speaker.
Transcript below
Join Community IT CEO Johan Hammerstrom and nonprofit AI expert Sarah Di Troia for a conversation about using Artificial Intelligence with ethics both within your organization as staff do their jobs, and with your community as you work with your partners, volunteers, and funders to achieve your mission.
Learn the basics about AI in the nonprofit workplace
Explore ethical questions surrounding AI. Nonprofits may be uniquely positioned to understand and draw attention to the impact of AI.
Learn how to craft organization policies around ethical AI use
AI tools are growing ahead of the capacity of governments, the private sector, and nonprofits to develop policies around the ethics of these tools. It is clear the marketplace is not going to slow down on introducing new applications of AI. Sarah Di Troia argues that the nonprofit sector is uniquely positioned to understand, evaluate, and publicize the impacts of AI on our communities, including our work communities.
Join Sarah and Community IT CEO Johan Hammerstrom for a deep discussion of AI applications and ethical questions, and learn to craft governance policies for your own nonprofit.
As with all our webinars, this presentation is appropriate for an audience of varied IT experience.
Community IT is proudly vendor-agnostic and our webinars cover a range of topics and discussions. Webinars are never a sales pitch, always a way to share our knowledge with our community. In this webinar we will be discussing a popular and common tool used by many nonprofits, and sharing our technical advice and insights based on what we are seeing among our clients and in the community.
Presenters:
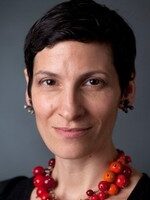
Sarah Di Troia is Senior Advisor at Project Evident and an expert in crafting strategy and operations to drive high growth in nonprofit and for profit organizations. Her experience as an investor, advisor and leader fuels an approach that integrates market insights with the internal change management necessary to realize new opportunities. Sarah is known for teasing order out of the creative chaos of growth and innovation. Prior to joining Project Evident, Sarah was Chief Operating Officer at Health Leads and a Managing Partner at New Profit, Inc. She earned her MBA from Harvard Business School.
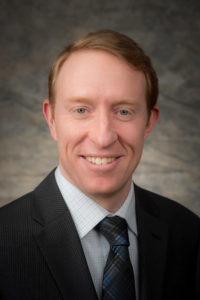
Johan Hammerstrom’s focus and expertise are in nonprofit IT leadership, governance practices, and nonprofit IT strategy. In addition to deep experience supporting hundreds of nonprofit clients for over 20 years, Johan has a technical background as a computer engineer and a strong servant-leadership style as the head of an employee-owned small service business. After advising and strategizing with nonprofit clients over the years, he has gained a wealth of insight into the budget and decision-making culture at nonprofits – a culture that enables creative IT management but can place constraints on strategies and implementation.
As CEO, Johan provides high-level direction and leadership in client partnerships. He also guides Community IT’s relationship to its Board and ESOP employee-owners. Johan is also instrumental in building a Community IT value of giving back to the sector by sharing resources and knowledge through free website materials, monthly webinars, and external speaking engagements.
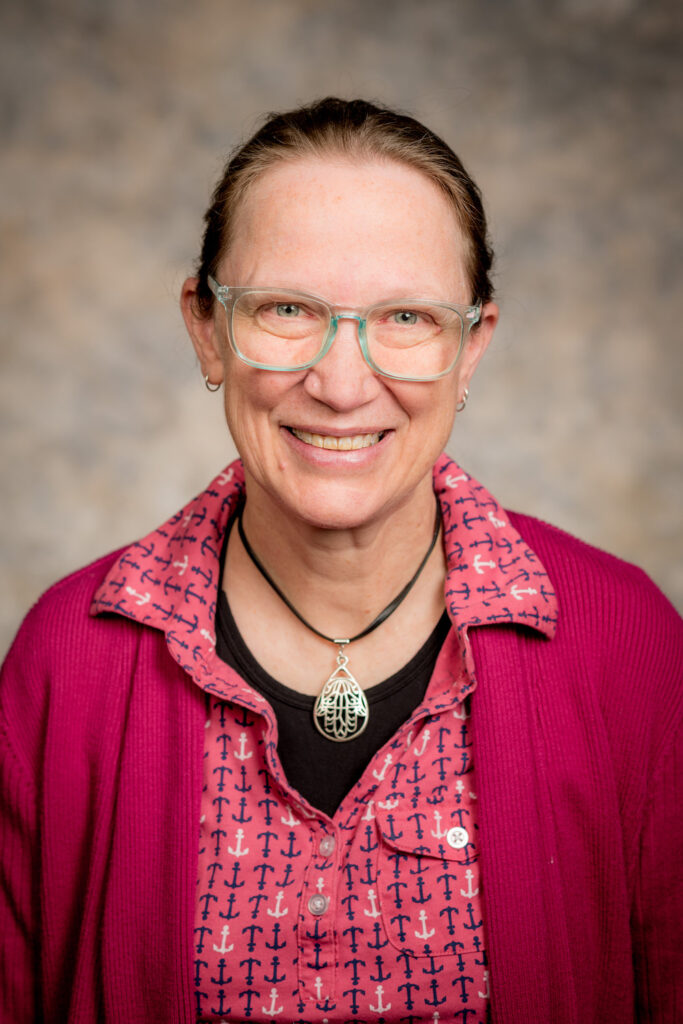
Carolyn Woodard is currently head of Marketing and Outreach at Community IT Innovators. She has served many roles at Community IT, from client to project manager to marketing. With over twenty years of experience in the nonprofit world, including as a nonprofit technology project manager and Director of IT at both large and small organizations, Carolyn knows the frustrations and delights of working with technology professionals, accidental techies, executives, and staff to deliver your organization’s mission and keep your IT infrastructure operating. She has a master’s degree in Nonprofit Management from Johns Hopkins University and received her undergraduate degree in English Literature from Williams College. She is happy to be moderating this webinar on Artificial Intelligence (AI) and Ethics for nonprofits.
Transcript
Some of the resources Sarah mentioned in the webinar:
Nonprofit AI Use Survey:
From the Stanford Social Innovation Review website:
“At the Stanford Institute for Human-Centered AI, we believe it is imperative to have diverse perspectives in building and understanding AI’s impact. That is why we have partnered with Project Evident, a nonprofit focused on data and evidence use in the social and education sectors, to better understand your needs and interests in AI. Together, we plan to co-design customized educational resources so [nonprofit] organizations like yours can effectively and ethically leverage AI to further your mission and impact.
But to do so, we need your help. Take this 10 minute survey to tell us about your current AI use, and learning needs. We will share our findings in a whitepaper in Winter ‘24. Together, drawing on Stanford’s leading research and your real-world expertise, we can shape more empowering, transparent, and equitable AI.”
This is a blog from Project Evident about philanthropy’s role with regard to getting involved in AI.
This is a press release about the partnership with TAG Technology Association of Grantmakers on an AI adoption framework for technology leaders at foundations.
Project Evident has three case studies about practitioners using AI:
A leader in equitable access to education leverages AI for impact
A leader in technology for education puts artificial intelligence into practice
Generating on-demand actionable evidence first place for youth and gemma services
Introduction: AI and Ethics for Nonprofits
Carolyn Woodard: Welcome everyone, to the Community IT Innovators’ presentation on Artificial Intelligence, AI, and Ethics for Nonprofits. I am really excited to introduce our panelists today. We have Johan Hammerstrom, who is the CEO of Community IT, and Sarah Di Troia, who is with Project Evident.
We know that artificial intelligence, or AI, is coming at us at a hundred miles an hour and a few years from now, it will have transformed our workplaces and our communities. So if you’re trying to use AI for your work, or if you’re worried about the impact that AI will have on the communities where you live, you are not alone.
Sarah Di Troia is at the forefront of considering the ethics of AI and how nonprofits can advocate for ethical use of AI.
My name is Carolyn Woodard. I’m the outreach director for Community IT, and I’ll be the moderator for today. I’m very happy to have our panelists here. So, Johan, would you like to introduce yourself?
Johan Hammerstrom: Yes. Good afternoon. Thank you, Carolyn. My name’s Johan Hammerstrom. I’m the CEO at Community IT. I’ve been with the company for over 20 years, have done a lot of work with nonprofits and helping them use technology effectively during that time. And over that 20 year period, I’ve seen a lot of new waves of technology coming into the sector and creating opportunities and also challenges for nonprofits. And it definitely feels like we’re starting to see something new with all of this AI technology.
I have a lot of questions about it, so I’m really excited that Sarah Di Troia is joining us today to share her wisdom and knowledge on AI and how nonprofits can use it. So, Sarah, can you introduce yourself?
Sarah Di Troia: It’d be a pleasure. Hi everyone, I’m Sarah Di Troia. I’m a strategic advisor with Project Evident. I’m focused on product innovation. I’ve been in the nonprofit sector for the last 30 years, both as a funder, as management leading COO, leading a scaling nonprofit and then as an independent consultant.
And in addition, in my work with Project Evident, I’ve spent the last three plus years just doing a deep dive on AI. Project Evident cares about practitioners, nonprofits, being able to own their own data and being able to use that data to drive their own learning agenda, so they can get better outcomes for their communities.
We look at AI as an incredible opportunity for nonprofits to be able to make a positive action with the data that they’re already collecting and to create more equitable outcomes for the communities that they serve through use of AI. So we’re really excited about the advent of AI and I spent the last three years doing AI research, leading cohorts of nonprofits, getting ready to pilot different types of machine learning applications.
Currently, Project Evident is partnered with the Stanford Center for Human-Centered AI. We are doing a national survey on nonprofit and foundation AI use, and I hope many of you’ll choose to take that survey so we have a robust understanding of what’s happening with AI. And I’m just really delighted to be in dialogue with Johan and dialogue with all of you about AI and where it could go.
Project Evident
Carolyn Woodard: Thank you, Sarah. Would you like to tell us a little bit more about Project Evident?
Sarah Di Troia: Thank you. And just to mention briefly on the survey, we want as many folks as possible inside your organization to take the survey, so if you choose to take it, please do pass it on. We’re hoping that we can do subgroup analysis so we understand how people in different types of roles, as well as different types of organizations in terms of the programmatic focus are using AI. So share broadly, share liberally.
Project Evident was founded because we watched how evaluation and data was being used as a way to sort of thumbs up or thumbs down on a program model as opposed to using data the way it’s used in the for-profit sector, the way we use it in organically in our own lives, which is to make better decisions and to learn. And so the goal was, how do we help nonprofits and practitioners and funders use data in a way that really fuels their own learning agendas?
What is it you’re trying to learn how to do with your community? How can data help you do that? And inform the action of management, the action of frontline staff and also inform the community that you serve ultimately leading to more equitable outcomes. So that’s the goal of Project Evident. The signature work that we do is the Equitable Recovery Wallet, which is free services around data and data analysis and use in systems for small nonprofits, early journey nonprofits, as well as strategic evidence planning. Think of it as a strategic plan for your evidence building with larger nonprofits. And then over the last several years, we’ve gotten involved with AI work as well.
Carolyn Woodard: Awesome. Thank you so much. I just dumped a whole bunch of links in the chat about Project Evident and their work (see links above.) So I really appreciate that Sarah.
Community IT
All right, so before we go much farther, I’m going to tell you a little bit more about Community IT. If you’re not familiar with us, we’re a 100% employee owned, managed services provider (MSP). We provide outsourced IT support and we work exclusively with nonprofit organizations. Our mission is to help nonprofits accomplish their missions through the effective use of technology. We’re big fans of what well-managed IT can do for your nonprofit.
We serve nonprofits across the United States, and we’ve been doing this for over 20 years, as Johan said. And we are technology experts. We are consistently given an MSP501 recognition for being a top MSP, which we received again in 2023. I want to remind everyone that for these presentations, Community IT is vendor agnostic, or in this case, maybe I should say tool agnostic. So we’re going to talk about a lot of different AI tools that are out there. We only make recommendations for our clients and only based on their specific business needs.
But you know, it’s in everybody’s interest to learn more about what’s out there, what the landscape is, and how these tools are working. So I want to go on to the learning objectives for today.
AI and Ethics Learning Objectives
We’re hoping that by the end of today, you’ll have learned
- what we mean when we talk about AI for nonprofits and
- be able to recognize some common AI applications. Some you may already be familiar with, some may be new to you.
- Discuss what organizations need to have in place before implementing AI.
- And Sarah is going to offer some examples of nonprofit sector usage of AI to enhance impact and equity.
Poll 1: Do you use AI?
But before we do that, we’re going to start off with a poll.
Does your organization use AI now?
So you can answer yes, no, you’re not sure or not applicable, so it’s not a trick question. We really just wanted to get a sense of where you are on this journey. And if you’ve never used it, you want to find out about it, you’ve come to the right place. If you’re already using it and kind of trying to figure out how to do governance and what it can really be used for, you’re also in the right place.
And there’s a good question, AI in general or generative AI? So we’re going to talk about that difference in just a moment. But go ahead and answer from your understanding, I think would be the easiest for this one [are you using any AI.]
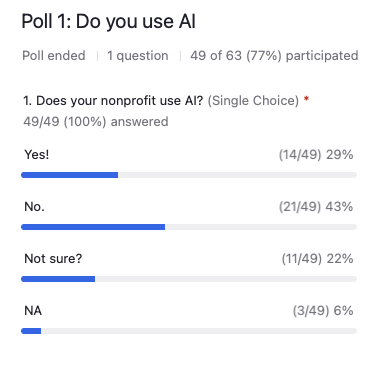
And Johan, would you like to read the results for us?
Johan Hammerstrom: Sure, just about 30% of respondents selected yes, their nonprofits are using AI. Just over 40% responded, no. Around 20% responded, not sure. And then the remainder said it didn’t apply to them. So it’s pretty mixed.
Carolyn Woodard: Excellent. Okay. So, and a little bit of a bell curve there.
AI and Generative AI
Sarah, then that is the perfect jumping off point for your explanation of what is the difference between AI and generative AI?
Sarah Di Troia: Sure. AI is a technology that allows you to mimic human cognitive function. So whether that is decision making or problem solving, essentially it mimics the way our brains help us do those types of tasks. And the way it does that is you feed it lots and lots of data and it learns from that data to predict what the answer to the problem might be using past data.
That’s different from generative AI. Generative AI is still predictive. But it’s trying to create something new. It’s not looking at a new use case, examining thousands of prior use cases or hundreds of use cases and then determining what’s a likely answer. Generative AI is trained on massive data sets, and it generates new speech in terms of sound. It generates new writing; that would be ChatGPT. It generates new visuals, so you can ask it to print an image. It can also create video.
So, generative AI uses large, large amounts of data. So folks might be aware that ChatGPT 3, not 4, which is also in the market, but 3 was trained using all of the available information on the internet up to 2021. So that was the training dataset, a pretty large dataset that was used to be able to make predictions about how to respond to questions. It’s still basically a predictive technology, but it’s generating new content, new pictures, new sound.
You probably have also heard the words machine learning and machine learning versus AI. This is essentially for lay people, the difference between ice cream and gelato. Like, yes, there’s a difference between these two delightful frozen treats that use sugar and cream and ice. But if you’re really hungry on a hot day and you want something sweet, either one will do.
Technically, machine learning applications are a subset of AI, not generative AI. A machine learning application would be something like a recommendation engine, which you all experience with Netflix or with Amazon when you make a purchase. Would you like to see this movie? Would you like this book? Or a virtual assistant, which you have probably encountered if you were trying to change your airline flight or maybe if you were shopping online and suddenly a virtual assistant asked if you needed help.
Or natural language processing: Hey Google, Hey Alexa, Hey Siri, the ability to understand language. So those are all different types of machine learning applications, and there are many more. By the way, those are just three that you probably have encountered in your day-to-day existence at this point.
Johan Hammerstrom: I really like that analogy of ice cream versus gelato. I think that really helps clarify what we are now calling AI and truly AI technologies that we’ve been living with for a while now.
Why AI Now?
One of the questions I have is why now? My understanding is that some of these generative AI models have been around for four or five years, and it seems like it all just sort of exploded onto the scene in the last year, and there’s a lot of hype around AI and AI tools. And I’m curious, what’s behind that and is that real or is this just part of the tech industry hype cycle?
Sarah Di Troia: Right, it does feel like we’ve been in a constant hype cycle for the last decade of different types of technologies that are going to revolutionize our world. So I want to talk a little bit about why now for traditional AI, some of those machine learning applications that I just referenced, as well as why now for generative AI.
Johan, you’re absolutely right. The antecedents of AI have been around for probably 25 years in terms of academic research. In terms of the commercialization of this technology, certainly some of those machine learning applications have been readily available from large companies for the last decade.
I mean, it’s transformed the way that we all interact as consumers with companies. We’re no longer in a one size fits all world in terms of the way you interact with an online retailer, sometimes even with an offline retailer if you have one of their credit cards.
So, what’s happened with the traditional AI is what happened with websites. So for those of you who were not around when the internet first came, suddenly everybody needed to create websites to do that. You went to a website design firm and there were graphic designers, there was a project manager, there was a copywriter, there was a coder. There was a whole team of folks that had different specific capabilities that would custom build a website for you.
Flash forward to today, and you could probably have a high school intern build a pretty decent website for your organization using Squarespace. You don’t need to have technical capabilities, you don’t need to have design capabilities. And you can probably even stand it up so that you can process credit card payments. So that moving from customization to a tool that a non-technical person can use, we travel that path with traditional AI.
Recommendation engines, chat bots, predictive analytics, there are now tools that are available to you through the Amazon Stage suite, through Microsoft that you can literally just use the package and you can upload your data and you can kind of drag and drop.
So I think the time is now for sort of traditional AI because it’s going to get really cheap and it’s going to get much more broadly available to folks. And you don’t need to have the same level of technical expertise to be able to program.
In terms of why now for generative AI, is the fact that it got released publicly. And some of our largest companies are search companies, Google’s now is a monopoly, in a legal situation in terms of its monopoly on search. Search is an incredibly lucrative industry, and I think one of the reasons why we’re in the hype cycle is that ChatGPT actually is a way to completely upend the way that we search things and the permeability of information. So that it is more integrated when we receive it, as opposed to looking at 12 different links and looking at lots of different information and then you doing the integration.
So, I think it’s possibly going to upend a big company, and that’s why there’s a bit of hype around it. And the fact that it was publicly released and it was a cool tool and it was free that certainly drove a lot of interest as well.
Johan Hammerstrom: That’s really interesting. I wonder if, because Microsoft obviously is a big investor in open AI and one of the first use cases for text-based generative AI was Bing. In some ways maybe Microsoft saw that as an opportunity to finally gain some search market share from Google. I’m not sure if that’s happening. But that’s interesting. It’s important, I think, to see the tech industry business behind the technology itself. The technology doesn’t exist in a vacuum, it’s being created by these very large corporations for very specific business purposes. It’s always good to be reminded of that.
Sarah Di Troia: That’s right.
Johan Hammerstrom: I think that’s a good segue into talking a little bit more about what nonprofits can do to be ready for AI, because all these tools are coming out, and I think there’s always the fear that if you don’t jump on the train quickly enough, it’s going to leave the station and you and your organization are going to be left behind. I think we have another poll before we get to that question.
Carolyn Woodard: Let’s get to that poll. All right. So we wanted to know from you, how your organization has addressed AI. When we were talking about this before with Sarah, when we were preparing for this webinar today, we came up with these different levels of where you are at in addressing AI.
Poll 2: How has your organization addressed AI?
- So the first option is we don’t have an AI policy or governance. It’s pretty much a free for all. So if you have a tool or are using a tool that has AI built into it, you just go ahead and use that as an employee.
- The next answer is we have an AI policy staff as individuals know how they are allowed to use AI to do their work. So you’ve started thinking about it, maybe you’ve put a policy together or some governance or are in the process of putting some governance documents together.
- Another layer is that you have tools that use AI and you’ve gone beyond just having governance documents, you’re intentional about increasing your efficiencies to increase your impact. So you’re looking for ways that you can use AI tools to make your organization work more efficiently and achieve your mission that way.
- The last level was embracing AI to achieve your mission in customized and intentional ways. So maybe even transformative ways of doing your mission perhaps differently because AI allows you to do it in a different way in the community where you work.
Johan Hammerstrom: So, the vast majority of respondents, 85% don’t have an AI use policy or governance. It’s a free for all. I’m not going to say that’s wonderful, but you’re in the right place. We are going to be talking about this exact issue and you’re not alone. So don’t feel bad if that’s the answer that you gave, because that’s 85% of the people on the webinar today.
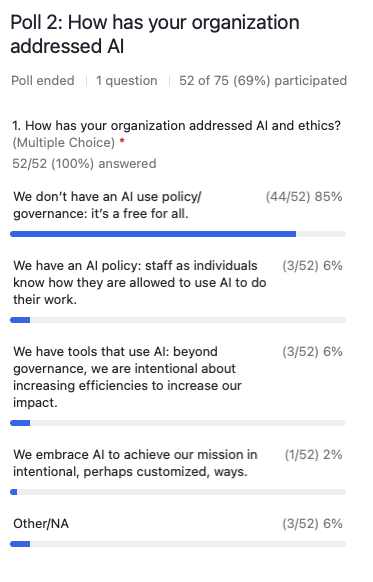
Six percent have an AI policy, 6% have tools that use AI and the remainder are non-applicable. Well, 2% were embracing AI to achieve their mission in intentional ways.
Carolyn Woodard: Very interesting to hear, and thank you everyone for sharing that with us. And thank you Johan, for mentioning you’re not alone. Everyone is struggling to figure out how to use this and how to have governance around it, which is why we have Sarah here with us today.
AI Readiness for Nonprofits
Okay. So I want to move on to our next topic which is around readiness for AI. So Johan, I think you had a couple questions for Sarah.
Johan Hammerstrom: Oh, lots of questions. One of the things that I have to keep reminding myself of, is that you can’t just deploy technology. You can’t just launch it. You have to prepare first. And it takes a lot of discipline to remind yourself of that, especially when they’re really interesting, novel technology tools being released. Our tendency is to kind of jump in and start using them and figuring out how they work. But there is a lot of important preparation that needs to take place first.
I’m really, really interested in hearing more about what Project Evident has been doing. You mentioned in your introduction that you’ve spent the last two years really deeply focused on AI and figuring out how it’s going to fit into the larger goals of Project Evident. So I’d love to hear more about what you’ve found in your research and how your findings can be used by nonprofit organizations in getting themselves ready for using AI tools appropriately.
Sarah Di Troia: Before we kicked off the webinar, I was chatting with Carolyn and Johan a little bit, and I just talked about how much I love moments of tremendous change, both inside organizations and across our sector. I find those to be really fertile, exciting moments, and we’re definitely in those moments right now, both inside our organizations and in our society at large. I have a lot of enthusiasm and excitement about what can happen and what may happen for us in the future.
One of the things I really have loved doing at Project Evident is, I’ve thought a lot about AI adoption inside organizations, and I’m going to focus on that initially and share some resources. I then want to talk a little bit about what I think some opportunities are for AI in the sector and maybe I’ll share some examples of that as well.
Johan, I really appreciate the way you framed this. There’s a lot we can do to get ready. And this became really apparent in the first cohort that I led, which was preparing a set of education organizations who specifically wanted to work with recognition and that was the ML (machine learning) technology that was of interest to them. And we thought we would start this cohort, day one of a six month cohort, was going to be, let’s talk about the problem that you want to focus on. That recommendation can be a smart solution. And we realized, oh, people were nowhere and near identifying the problem, and actually we need to start earlier. And that really became the basis of working with that group.
AI Readiness Diagnostic for Nonprofits
Then doing a bunch of masterclass webinars around AI readiness morphed into what we call the AI Readiness Diagnostic. That’s on the Project Evident website. It’s a diagnostic of 12 questions. I’m going to give you a high level of the four buckets that falls in.
And when you go through the diagnostic, which we highly recommend that you do with program technology and your measurement and evaluation on learning staff. No one person has all of this information in your organization, as it should be because AI projects are across silos inside of your organization and really require collaboration. But the diagnostic will then give you a customized report with additional resources and some ideas about how to prioritize and help you interpret your scores.
- Not surprisingly, the place that we really would love you to start is with equity and design justice.
So if you are in an organization that has never brought end users into a design process, that’s something you should learn about before you jump into building an AI pilot. If you haven’t done your DEI work to be able to talk about difference and equity, often for organizations that deploy an AI tool, they will learn that maybe there were some inequities around how their program was being delivered to different subpopulations of clients that they work with. Being able to contextualize that and talk about that and learn from that is really important. So design for justice in equity is the most important that you have some basics around some skills and some work you’ve done as an organization.
- The next is strategic purpose.
If you’re going to undertake a large project, you want to make sure that your board and your leadership is on board.
- You also have to have real clarity about your program logic model.
The secret of using AI in the nonprofit sector is that your program logic model is the algorithm, all of that hard won knowledge about how you create change for somebody that is actually the algorithm that you’re seeking to animate. And so having a really clear program logic model that reflects what is actually happening on the ground, not what we told funders, not what was happening three years ago. It really has to reflect the best of the knowledge of your frontline staff.
- We then ask people to really focus on knowledge and skills.
What are the capabilities that you can either have on staff or can access on staff?
- And then we talk about data and systems.
Where’s your data stored? How can it be accessed? What is the quality of your data? What’s the amount of the data that you have?
So designed for justice and equity, strategic purpose, knowledge of skills and systems and data. Those are the things you can do in advance. Frankly, you need to do them in advance to have a strong foundation on which to stand to be able to build an AI pilot. I’m going to pause there, Johan, and see where do you want to go next?
Johan Hammerstrom: Yeah, it occurs to me that those activities require a lot of reflection by the organization. The impression I’m getting is that these tools are about augmenting the intelligence that the organization already has and enhancing their capacity to do what they’re already doing and maybe creating new opportunities and new ways for them to do that.
Program Logic Models
I’m wondering, particularly with the logic model, how technical does that need to be? Is that something where the organization really needs to understand how the AI tool is going to be implemented in order for them to carry out that work?
Sarah Di Troia: The program logic model, and there are lots of great resources online, just type in “program logic model,” and lots of templates will show up. Or you could ask ChatGPT for the best program, create the most integrated best program logic model for you.
What’s important about the program logic model is it doesn’t have to be technical, it has to be representative. So it actually has to represent the different steps that move from inputs to activities to outcomes, to long-term change that you believe you’re having, not at a societal level, but at an individual personal level.
That’s what has to be reflected, because within that, you can begin looking at it with your community, again, this is design justice, right? With your frontline staff, with your community, and say, in some aspect of this program logic model, if some step in this program logic model, if you knew more, could you do better? Could you get better, more equitable outcomes?
And that’s going to help isolate the places where the folks who have the most lived experience, the most lived expertise are going to be able to identify for you. That’s the problem we’re trying to solve within our program logic model.
Doing better here might be precision analytics. If we could have better subgroup breakdown so we knew what elements of our program model best served different types of our clients. It could be a recommendation engine. If I was better able to connect more teachers with a particular professional development that was just in time, perfectly matched that would support them on being better prepared in their classrooms.
The program logic model can be agnostic of the type of machine learning application, but the program logic model will be your map to help understand what is the problem you’re trying to solve. And then you can figure out if one of the tools that are in the market can help you solve that problem.
AI and Ethics and Your Data
Johan Hammerstrom: Got it. That’s very helpful. One of the sort of aha moments for me in hearing you talk about AI and preparing for this webinar is the degree to which this is also data driven. You mentioned that ChatGPT I believe was trained on all of the data on the internet, which is kind of an inconceivably massive amount of data.
But, the impression that I’m getting is for nonprofits to get ready to use AI tools effectively, they also need to be aware of their own data because it’s really their data that’s going to be informing the AI tools that they start using.
Sarah Di Troia: So here’s what’s really exciting about using AI tools, absolutely. Some of the data that you’re using is your data. And by the way, you don’t need all the data from the internet up to 2021 to inform your recommendation engine.
For recommendation engines, you probably need about 200, 250 quality data cases. And I’ll explain a little bit what that means. So I just want to right size the expectation. The other really interesting thing though is that in the last decade, our government has been releasing more and more data sets to researchers and just making them available to the public. So you have the ability to now integrate your data with census data, which can be incredibly rich data.
AI Ethics In Practice: Equal Opportunity Schools
There’s a school that is using a recommendation engine to identify students who are overlooked for AP or IB classes. It’s called Equal Opportunity Schools. That’s always been their program model, but they’ve done that by achievement and they’ve had a human look at school-based data: attendance, grade point average, their own survey data that they would collect inside the school, as well as some types of data that is outside in the community. They’re now using a recommendation engine to bring census data, school data, as well as their own collected data to then recommend which students are being overlooked.
Now, importantly, that recommendation is not then just being given to the school. The recommendation is being given to the coach, the frontline staff person who it was always part of their job to find those overlooked students. They now just have a machine learning application, a recommendation engine that’s making those recommendations to them for them to verify. It frees up their time, so that they can be more efficient in their decision making and spend more of their time focused on making sure that the students who do end up in those classes are successful, which is the other part of their job.
Johan Hammerstrom: That’s such a great example, because it really shows how these tools can enhance the human intelligence that’s needed to do this work. And it also sounds like they were very intentional about the approach that they took in, in developing that system. How long did it take? What was their overall process? How long did it take for them to get ready? What led them to deploying and implementing those tools? What were some of the challenges that they ran into along the way?
Sarah Di Troia: First of all, Equal Opportunity Schools was part of one of the cohorts that we ran. I think what was most exciting is that it’s a relatively large nonprofit and that you had a frontline coach person, somebody in that role talking to somebody who did measurement, evaluation and learning as well as technology.
So when we ran that cohort, we said, you need a representative from each of these plus somebody from senior management that’s willing to be in collaboration with each other, understanding what the problem is and beginning to design what that solution could look like. I think one of the most exciting things for us was when the measurement evaluation and learning person said, I didn’t know this person on staff, and now she’s my go-to as somebody who I want to talk to at least once a week to help me make sense of information that I’m receiving from across the network.
So, I think one of the things that’s really powerful about these projects is that they have an opportunity, they have a necessity frankly of knitting together. It’s not a technology project. I know I’m talking to the Community IT community but it’s not a technology project. If you want to use AI to drive your outcomes, to attain your mission, this is a program project that is supported by evaluation and measurement and learning and supported by technology. All of you have to be on the same side of the table together, working together.
The challenges that they faced was, how do we engage the rest of our organization on this because we have some fear that we’re going to overlook students by going in this direction versus having it still be done by a human.
Change Management
So like any big shift that you’re doing inside of your organization, there was a change management activity that had to happen. And that activity was making sure people understood the technology, understood the piloting that would happen in a safe space. So they could use data from prior years to run basically the same analysis that people would run as individuals to see whether or not the recommendation engine would be as strong as having a person do it.
It was, how do I bring my organization along? That’s training, that’s learning, that’s transparency. And also, how do I keep the mission front and center? Because the goal was, we want to serve more kids, we want to serve more students. If we’re going to be tied to a human being looking at all the data to identify those students, then there’s a limit to how much money we can raise and how many students we can identify. But if the identification is the bottleneck, not the ensuring they’re on the right track, and you can release that bottleneck, well, suddenly I can achieve more of my mission.
AI and Ethics at Nonprofits
I think when you put the mission front and center, that really helps people change because we’re all in it for the mission. We could all be doing something else and probably making more money. We’re all here for the mission. I think keeping that front and center and making sure that you’re emphasizing equity and not being biased goes a long way to bringing organizations along.
Johan Hammerstrom: It’s an amazing example. I loved hearing about that organization and the approach that they took, and I think one of the things that really stood out to me is that it was so human centered and human focused. And certainly the mission focus keeps recentering them on the human need and the human objective.
I think one of the big challenges that we all face, not just in nonprofits, but in society as a whole, is the fear that these tools are going to replace humans. And certainly, a big motivator behind a lot of the labor strikes now is this fear that the objective of the tool is to generate profit, not to solve human problems. There’s just such a wonderful opportunity for nonprofits to use these tools in a very different way and really become an example for how these tools can help society rather than enriching small segments of society.
Sarah Di Troia: One of the challenges is that these tools have been really developed and honed by the commercial sector, which means profitability and scalability are the two most important design criteria. And you know ethics and equity, that’s not how those organizations are incentivized or compensated.
I think one of the powerful things we can do as a sector is get involved with AI. Even though it’s not perfect, because if we wait for it to be perfect, we’ll never be a part of it. We’ll definitely make mistakes because that’s the only way people learn is to make mistakes. But if we start demanding tools that are not biased and have equity at the center of their design, that market pool is going to eventually exert influence on the market. And so I think getting involved is one of the ways that we shape the market.
Data Bias and Ethics Issues
Johan Hammerstrom: And I think in order to be able to do that, it definitely helps to understand some of the problems or challenges that exist with the current tools in terms of their data biases, the ethical issues that are inherent to how the tools have been built. So I was wondering if you could talk a little bit more about that?
Sarah Di Troia: Yeah, absolutely. First of all, I just want to be clear that all data and all models have bias. I love that we’re talking about AI and bias. It’s so important because of the scalability of technology. But I want to bring this same level of focus for whenever we’re talking about data and whenever we’re talking about models, data and models are collected and crafted by human beings. We’re creatures of bias. Our bias is reflected in those tools.
Where you see bias come in AI is in two places,
- one is the dataset on which something is trained,
- and then the second is the algorithmic model that underpins the application.
AI Bias – the Data
We’ve talked a bunch about ChatGPT, we’ve talked about the fact that it was trained on all the data on the internet up to 2021. How much of the data on the internet is from the global south versus the global north? I mean, there’s pretty European, American, global north-centered information on the internet relative to other countries and other parts of the globe. So you can think about the bias that already exists in ChatGPT just around global north, global south, let alone other -isms that are a reflection of our society that have been magnified or maybe just mirrored on the internet.
So if you want to hire more women and you’re using a data set to try and figure out who is successful and likely to get promoted and all your data is showing that men get promoted inside your organization, if you use that as your training data, as a way of figuring out which resumes that you should look at that are going to be recommended to you, it’s going to not recommend that you see any female resumes because you’ve just taught the algorithm by the dataset that that is not the type of person that can be successful in your organization.
Even if your intent at the beginning was to diversify your organization, who is in and who is not in your dataset is incredibly important in terms of training your algorithm.
AI Bias – the Algorithm
The second place where bias comes in is just the algorithm itself. Now, rarely is the algorithm saying we don’t want women, we don’t want Catholics, we don’t want X, Y, Z, right? That’s not likely what’s happening. What’s happening is that the algorithm is valuing something that is more likely to be associated with a race, an ethnicity, a religion, or a gender.
A really interesting case study is from Women’s World Banking. They were looking at algorithms that were being used to decide who was getting micro loans by different micro lending and banks globally, they noticed that women were not getting as many of these loans.
And what they saw in the algorithm is that when it was weighting prior work experience, retail work experience or work experience in the home was not weighted as highly as work experience in an office or work experience on a job site. So because the weighting was different and women had a greater propensity to have retail experience or work in the home, they were not getting nearly as many of the loans because they were not deemed as credit worthy.
It didn’t say we favor men over women, it was something else that was in the algorithm. So often there’s a hunt to figure out what you put in your algorithm that is actually telegraphing a bias because it’s correlated to a certain subgroup of people.
Identify AI Algorithm Bias
Johan Hammerstrom: And how do you identify those biases? Just through testing the algorithm, or are there other ways?
Sarah Di Troia: A couple different ways. First of all, if you’re going to buy an ML tool or work with somebody around an ML (machine learning) tool, you can ask questions like, what dataset was this trained on?
I want the evidence that this was trained on a full dataset that had subgroups that matter to me that were well represented, maybe didn’t include some information that I don’t want to have represented. So you can ask about what data was actually used for training.
On the algorithms, they have to be bias tested. It’s harder to do if you’re talking to Microsoft Copilot or huge companies around what they did. It’s going to be hard for us to get much purchase around getting feedback on that. But you can have the algorithms tested for bias. And then, algorithms are not a set it and forget it activity.
So, if you do have an algorithm that’s inside your organization that you’ve had custom built. You’ve trained it on old data, but every new piece of data that comes through is fuel, it’s learning for that algorithm.
So algorithms can drift because they’re sort of live creations with every piece of information that comes through them. So you have to be really vigilant on continuing to test and watch your algorithms to make sure they’re giving the responses that make sense for your populations and your values.
Johan Hammerstrom: That’s so interesting. It’s kind of a whole new way of thinking about technology tools. The idea that they evolve over time, that’s kind of a new way of thinking about it for me at least.
My impression has always been, well, this is a spreadsheet and this is what it does, and it just calculates all the numbers and that’s it. So it’s interesting to think about technology in this way.
Q&A
I could keep asking you questions for two more hours, Sarah, but I don’t want to monopolize the webinar here today. We’ve got some great questions coming in.
AI and Ethics: Policies
The first question is about AI policy. What kind of policies should nonprofits have? Are there templates that you would recommend nonprofits using?
And then the second part of the question is if there are additional risks beyond the ones we’ve talked about to nonprofits using AI and how those risks can be mitigated?
Sarah Di Troia: At a minimum, folks need to have an AI policy in place just for your team who is using ChatGPT, maybe using Otter.ai, and might be uploading documents into Grammarly.
What’s important for you as an organization is an agreement across the staff or with the executive team of
- what is open information that’s shareable,
- what is private information, but it’s shareable because it’s been de-identified.
- And then what is frankly either personal information or intellectual property, which cannot be shared.
And sharing is as simple as “I uploaded it into ChatGPT to get a good summary of this or to compare it to other models that are out there in the world.” If you upload something into ChatGPT, or if you invite Otter.ai into your meeting, the fine print on both of those tools is that your information becomes part of their fuel.
It becomes part of their training data. It also becomes part of the data that they can use to respond to new questions, new inquiries that come their way.
When ChatGPT came out, there were a bunch of news stories about companies that had uploaded customer data, they had uploaded intellectual property. And folks didn’t realize that they were essentially taking stuff that is not shareable in their organization and making it shareable to everybody. There are a bunch of tools out there that don’t require to officially be a part of your tech stack. They’re just available to consumers.
Really important to have a policy around how people might be using those tools to decrease their workload, and what data is shareable and what data really isn’t shareable.
AI and the IT Department
Johan Hammerstrom: It’s amazing, that takes the whole concept of shadow IT to a whole new level, that AI in your stack and AI outside of your stack, and really thinking about your stack, your data. It’s a great conceptual model for thinking about where all these things are going in IT. I’ll restrain myself from asking more questions and ask another question from our audience.
What human capacity resources do you recommend? Some level of information management leadership for policy development, process and tech adoption with the development of an internal data science analyst office that would support program staff to help advance the outcomes of their logic models? It’s kind of like a whole new way of thinking about the IT department. What recommendations do you have?
Sarah Di Troia: It depends on your strategy, depends on your goals. So if you are a nonprofit, like Crisis Text Line, Talking Points, will.org, these are all organizations that they’re AI natives. They were founded with their program model that has AI embedded in it. Those organizations have data scientists on staff. They have to have a technology team that’s doing internally facing work, but they have to have a technology team that’s just focused on their algorithms and the technology they’re using. That’s the core of their program model.
They also have to have a data scientist on their measurement, evaluation and learning team, right? Because the amount of data and the ability to analyze that data is radically different. If you are an AI native centered organization, that’s really different than if you’re a community-based nonprofit that wants to incorporate ChatGPT into your fundraising. You know, you can probably ask ChatGPT, what’s a good policy for fundraisers around AI. By the way, there is going to be an upcoming conference called Fundraising AI. That’s going to be a two day conference that’s free. That’s just all about fundraising and AI, because I know that often comes up for folks.
Advisory Committee
So it really depends on what’s your purpose, what’s your goal. I recommend, for nonprofits that are just getting involved, to create advisory committees. Look for a board member that has expertise that can join your board. They can help you with some of the operational pieces before you decide this is going to be a significant part of who we are and we have to have our own embedded staff.
And again, it all depends on what you’re trying to do. The tools are going to increasingly become drag and drop. That can be complicated because those tools are a black box. You may not know the data that they were trained on. You may not know whether or not the algorithm matches your values, but those assets are going to be available to you. And increasingly there are good algorithms that are available through GitHub, that are just open. And the development of algorithms is becoming more Lego-like versus custom coding. Even the cost of developing custom algorithms is coming down. So it’s going to come in your direction.
But the question is, what do you want to do with it? And how much is it going to be at the center of what you’re planning to do as an organization?
Johan Hammerstrom: And I would hope that there are sector-wide incentives for making sure those black box tools are either more transparent or ensuring that they don’t have bias. I could just see the misuse of some of these tools really undermining objectives that organizations are pursuing.
Sarah Di Troia: And this is one of the reasons why I think it’s so important that I know Carolyn shared a blog that I wrote with the Center for Effective Philanthropy, that was specifically about philanthropy getting involved. But I believe the same is true for nonprofits.
I think if we stand on the sidelines while AI technology is being developed, we won’t have a chance to make sure that equity and bias are really attended to in product development, because nobody else is asking for that right now.
– Sarah Di Troia, Project Evident
I won’t say nobody else is asking for it. Nobody’s getting paid to deliver it right now.
Carolyn Woodard: I wanted to just say thank you so much, Sarah, for all of this, and for those of you that have a couple more questions in the Q&A and all the great questions we got at registration, we’re going to try and answer all of them and also try to put all of these great links and resources that Sarah’s been mentioning today in the transcript.
Learning Objectives: AI and Ethics for Nonprofits
I feel like we hit all of our learning objectives:
- learning what we mean when we talk about AI for nonprofits,
- recognizing common AI applications,
- discussing what organizations need to have in place before implementing AI and
- offering examples of nonprofit sector usage of AI. You know, you had some great stories there.
I also want to make sure that I hit on our upcoming webinar next month, which is going to be October 18th.
We have an expert from Build Consulting, who’s going to talk about data and databases. So we’ll get a little bit farther into that question. And then our cybersecurity expert, Matt Eshleman, is going to join him. And we’re going to talk about the security that you need around data and databases, maybe in addition to, or different from your general cybersecurity that you have for all of your IT.
I’m just looking forward to it given this great discussion today on large databases and algorithms and models to get a little bit more into that.
You can also register for upcoming webinars here on our website, because even if you can’t attend in person, you can get on the mailing list. You can ask your questions at registration and suggest topics for future webinars for us.
So I just wanted to ask Sarah, before you go,
Final Q&A
There were a couple of questions about templates. I’ve been looking too, I feel like there’s no great resources out there yet with policy templates, is that true?
Sarah Di Troia: So how about if I commit to doing a little bit of research, so that when the transcript goes out, I’ll have some links for you? I was looking at them while we were talking and they’re behind a paywall. The Technology Association of Grantmakers has [templates if you are a member.]
I will say in developing the AI Readiness Diagnostic, it’s pretty incredible what you can ask ChatGPT to create for you. So one option is to do multiple iterative asks of ChatGPT to create a policy. But let me see if I can find some example links for folks as well.
Carolyn Woodard: That sounds great, because when I was poking around as well before this webinar, it seems like there’s a lot of very, very generic templates out there that maybe aren’t very geared toward nonprofits or the way nonprofits would want to have that policy in place. So that would just be incredibly useful.
The other question that we’ve gotten a couple times is the risks. The risks to nonprofits, maybe risks if you don’t have a policy, risks if you’re using AI?
Sarah Di Troia: I think there are two risks for folks who are just stepping into this.
- One is not having a policy that’s well understood by your entire team about what is shareable information. What is shareable, but has to be de-identified information? And then what is unshareable? It is intellectual property or it is personal information. I think that’s just incredibly important for folks to know.
- The second risk is increasingly AI is just going to be part of your tech stack. So there are some places where you might be asked to upgrade something and pay an extra fee to be able to have this AI based feature. And there are other places where AI is just going to be a part of things. And so I really encourage folks to ask their vendors, where is AI in the current product? And where is it going to be in the future versions of the product? So you are not caught off guard around your data being used when you haven’t even really consented to that.
I think those are the two risks for folks who are not proactively going out and trying to build algorithms, that you really have some control over saying yes or no.
Carolyn Woodard: I just want to thank you Sarah, so much for sharing all this expertise with us and giving us a lot of things to think about, a lot of resources to look into.
Johan, thank you too, so much for your time and for being able to interview Sarah today. And thank you everyone who attended and gave us all these great questions at registration.